A deep dive into the strengths, weaknesses, and unique features of n8n and Make.comβs AI agents, helping you choose the best tool for automation.. Ai Tools, Ai Automations.Β
Table of Contents
Introduction: The AI Agent Revolution in Automation
The automation world is going through a major makeover. What used to be simple tools for connecting apps, like Make.com and n8n, are now becoming AI-driven powerhouses with intelligent agents. It’s no longer just about automating tasks – we’re talking smart, adaptive workflows that can think for themselves.
After a lot of hype, Make.com finally stepped into the AI agent ring, going head-to-head with n8n, which has already been making moves in this space since mid-2024. The rapid adoption of n8nβs AI features definitely pushed Make.com to speed things up, making this a showdown worth watching.
In this comparison, weβll break down what each platform brings to the table. Weβll dive into the strengths, weaknesses, and key differences between Make.com and n8nβs AI agents. It’s not just about who’s leading right now β it’s about which one gives you the best foundation and future potential for your needs.
Ready to pick the right platform for your AI-powered automation strategy? Letβs dig in!.
Quick Summary: Comparison between n8n and Make.com
For those short on time: n8n currently holds a lead in dedicated AI agent features, leveraging its nearly year-long development advantage. However, Make.com is rapidly iterating and is expected to integrate similar functionalities, drawing inspiration from established patterns.
The core philosophies of the platforms remain evident in their AI implementations:
-
Make.com: Prioritizes a streamlined user experience and accessibility, catering well to users who value simplicity and a vast integration library.
-
n8n: Emphasizes flexibility, power, and customization for users with more technical requirements, while still striving for usability.
Now, let’s explore the detailed feature comparison, using this key:
-
π’Β Clear Current Advantage: One platform currently excels significantly in this area.
-
π΄Β Clear Current Disadvantage: One platform currently lags significantly.
-
π‘Β Competitive Area / Evolving: Differences exist, but the gap is narrower or likely to close as features mature or are adopted by the other platform.
Feature-by-Feature Comparison
1. Tools Attached to AgentsΒ π΄ (Make.com Disadvantage)
n8n: Offers a direct, intuitive method for attaching tools (other nodes or workflows) directly within the AI agent configuration. This allows for rapid setup of simple agentic workflows and facilitates complex structures like sub-agent communication (agents calling other agents or workflows).

Make.com: Relies exclusively on invoking separate scenarios (sub-workflows) for tool functionality. Each tool requires its own scenario, adding setup steps and potentially complexity, especially for agents needing multiple tools.

β Conclusion: n8n π’. Its approach is more integrated and flexible for defining agent capabilities. Make.com‘s reliance on separate scenarios reflects its linear workflow architecture, making n8n’s model difficult to replicate without fundamental changes.
2. Built-in Chat InterfaceΒ π΄ (Make.com Disadvantage)
n8n: Includes a built-in chat interface specifically for testing AI agents. This enables instant interaction and rapid prototyping, significantly speeding up the development and debugging cycle. You can test agent responses and tool usage immediately.

Make.com: Lacks a dedicated internal chat/testing interface for its AI agent module. Testing requires connecting to external triggers and chat platforms (Telegram, Messenger, etc.), adding friction and setup time to the development process.

β Conclusion:Β n8n π’. The integrated testing environment is a major advantage for efficient agent development.
3. Time to BuildΒ π‘ (Competitive Area / Trade-offs)
Make.com: Initial setup for AI agent subworkflows can feel slower due to defining scenario inputs, parameters, and triggers for each tool. However, its massive library (2,000+ integrations) is a significant accelerator, often providing pre-built connections that might require custom API work in n8n.

n8n: Agent setup itself can be faster thanks to direct tool attachment and the built-in chat. However, its smaller integration library (~1680 native integrations at last count) means users frequently need to build custom HTTP requests or nodes for unsupported services, which can be time-consuming and require API expertise. For example, attempting a complex integration like Pinterest might involve hours of exploring API docs in n8n, only to hit limitations, whereas Make.com might have a ready-to-use module.

β Conclusion: Mixed π‘. Each platform presents different potential time sinks. Make.com‘s vast integration library saves time connecting services, but requires more setup per tool within the agent context. n8n‘s quicker agent setup can be offset by the need for manual API work if required integrations are missing.
Learn How to Make AI Work For You!
Transform your AI skills with the AI Fire Academy Premium Plan β FREE for 14 days! Gain instant access to 500+ AI workflows, advanced tutorials, exclusive case studies, and unbeatable discounts. No risks, cancel anytime.
Start Your Free Trial Today >>
4. Vector Database SupportΒ π‘ (Evolving)
n8n: Provides robust native support for popular vector databases (e.g., Pinecone, Qdrant). This is crucial for implementing Retrieval-Augmented Generation (RAG), which allows agents to query internal knowledge bases. This underpins key business use cases like intelligent customer support and knowledge management chatbots.

Make.com: Currently lacks native vector database integration within its AI agent tooling.
β Conclusion: n8n π’ for now. However, vector database support is rapidly becoming table stakes for serious AI applications. It’s highly probable Make.com will add this capability soon to remain competitive.
5. Model Context Protocol (MCP) SupportΒ π‘ (Evolving)
n8n: Has introduced early native support for the Model Context Protocol. This emerging standard allows AI models to more intelligently understand the context and determine which tool/function to call based on natural language input, potentially simplifying workflow logic. Its current utility is developing but shows promise.

Make.com: Does not currently feature native MCP support.
β Conclusion:Β n8n π’ has the early advantage. As MCP gains traction and proves its value, it’s likely to become an industry standard feature that Make.com will also adopt.
6. Global AI Agents / ReusabilityΒ π‘ (Evolving)
Make.com: Offers the concept of templated AI agents (“AI Assistants”) that can potentially be reused across different scenarios. This could offer efficiency gains when deploying similar agent functionalities in multiple workflows.

n8n: Currently lacks a dedicated “global” or reusable agent template feature; agents are typically defined within the specific workflow where they are used.
β Conclusion:Β Make.comΒ π’ offers this specific feature. However, the practical, widespread advantage for most users is still emerging. For many, defining agents within their specific workflow context remains intuitive.
7. Memory StoresΒ π‘ (Competitive Area / User Need Dependent)
n8n: Provides multiple options for configuring agent memory (how agents retain conversation history). This offers greater flexibility for advanced users building complex, stateful interactions requiring different memory strategies.

Make.com: Offers more basic memory configuration options within its agent setup.
β Conclusion:Β n8n π’ for advanced users needing fine-grained control over agent memory. For many standard use cases, Make.com‘s options are sufficient.
8. Defining Parameters (for Subworkflows/Tools)Β π’ (Make.com Advantage)
Make.com: When calling a subworkflow (scenario) as a tool, parameters generally need to be defined primarily within the called scenario’s structure. Make.com intelligently maps inputs.

n8n: Often requires defining parameters twice: once when configuring the tool call within the main agent workflow (specifying what to send) and again within the subworkflow (defining what to receive).

β Conclusion:Β Make.comΒ π’ offers a slightly cleaner, less redundant process for handling parameters passed to subworkflows used as tools.
9. Testing Subagents / ToolsΒ π΄ (Make.com Disadvantage)
n8n: Excels here with features like pinned data (reusing previous execution data) and mock data capabilities within subworkflows. This allows developers to test tool execution in isolation without triggering the entire agent flow, significantly accelerating debugging and refinement.

Make.com: Lacks comparable built-in features for easily testing subworkflows (scenarios) in the context of an AI agent call. Testing usually involves running the full agent flow via an external trigger or building separate testing setups.
βConclusion:Β n8nΒ π’ provides a superior developer experience for testing the individual components (tools/subagents) of an AI agent system.
10. AI Model Choice & FlexibilityΒ π‘ (Competitive Area / Evolving)
n8n: Generally offers greater flexibility, allowing users to connect to various LLM providers (OpenAI, Anthropic, Google Gemini, Azure OpenAI) and often exposing more model parameters (like temperature, top_p) for fine-tuning. Its HTTP Request node also allows connecting to virtually any custom or open-source model endpoint. Β

Make.com: Tends to offer more curated, tightly integrated support for major LLM providers, prioritizing ease of use. While likely supporting popular models (GPT-4, Claude 3, etc.), the range of choices and exposed configuration parameters might be more limited initially compared to n8n‘s flexibility.

β Conclusion:Β n8n π’ currently leans towards greater flexibility for users wanting specific models or granular control. Make.comΒ π‘ likely offers simpler, more guided choices suitable for many users. This gap may narrow as Make expands its integrations.
11. Cost & Pricing StructureΒ π‘ (Competitive Area / Complex Trade-offs)
n8n: Pricing varies significantly with hosting.
-
Self-Hosted: Users bear direct LLM API costs (pay-per-token to OpenAI, Anthropic, etc.) plus their own infrastructure costs. This offers potential cost savings at high volume but requires technical management.
-
n8n Cloud: Typically involves platform costs plus potentially specific charges based on AI node executions or token consumption, bundled into their tiered plans.

Make.com: Integrates AI usage costs into its existing operational credit system or specific plan tiers. Pricing is generally easier to predict within their ecosystem, but might abstract away the granular token costs, potentially leading to higher perceived costs for very heavy usage compared to direct API access.

β Conclusion:Β π‘ Highly dependent on usage scale and hosting preference. n8n (self-hosted) offers potential for the lowest direct cost but adds complexity. Make.com provides simpler, integrated pricing within its platform credits, which is easier for budget forecasting but potentially less optimized for massive token volumes.
12. AI-Specific Error Handling & DebuggingΒ π‘ (Evolving)
n8n: Provides detailed execution logs where users can often inspect the input/output of nodes, including data passed to/from AI models. Error handling typically relies on standard workflow logic (error branches). Debugging might involve closely examining node inputs/outputs and potentially adding logging steps. Β

Make.com: Offers strong visual debugging for workflows. For AI agents, it likely shows successful/failed tool calls within the execution history. Inspecting the exact LLM prompt/response or handling nuanced AI errors (e.g., poorly formatted JSON output from a model) might require specific configurations or might be more abstracted initially.

β Conclusion:Β π‘ Both platforms are evolving here. n8n might offer easier access to raw data for technical debugging. Make.com‘s visual approach might be more intuitive for standard errors but potentially less transparent for deep AI-specific issues initially. Maturity in robust AI error handling (like automatic retries on transient LLM errors, specific parsing logic for model outputs) is a key area to watch.
13. Monitoring & Observability (AI Usage)Β π‘ (Competitive Area / Platform Dependent)
n8n:
-
Self-Hosted: Requires users to set up their own monitoring solutions (e.g., logging platforms, metrics dashboards like Grafana) to track agent performance, token usage, and costs. Offers maximum flexibility but requires effort.
-
n8n Cloud: Likely provides some built-in dashboards for overall workflow execution counts and potentially basic AI step usage, integrated into the platform UI.

Make.com: Typically excels at providing integrated monitoring within its cloud platform. Expect dashboards showing operation consumption (which would include AI agent runs/tool calls), execution history, and potentially specific analytics related to AI usage and costs, all within the Make interface.

β Conclusion:Β Make.comΒ π’ likely offers better out-of-the-box, integrated monitoring within its ecosystem. n8n π‘ offers greater potential for deep, customized monitoring via self-hosting and external tools, but requires setup. For ease of monitoring standard usage, Make likely has an edge.
Business Use Cases: Where Each Platform Shines
The most impactful enterprise applications for these AI agents currently revolve around:
1. RAG (Retrieval-Augmented Generation) Systems:
-
Function as an intelligent knowledge base or “digital brain” for internal company information.
-
Power sophisticated customer service chatbots that can access and synthesize company documentation.
-
Current Leader:Β n8n (due to essential Vector DB support).
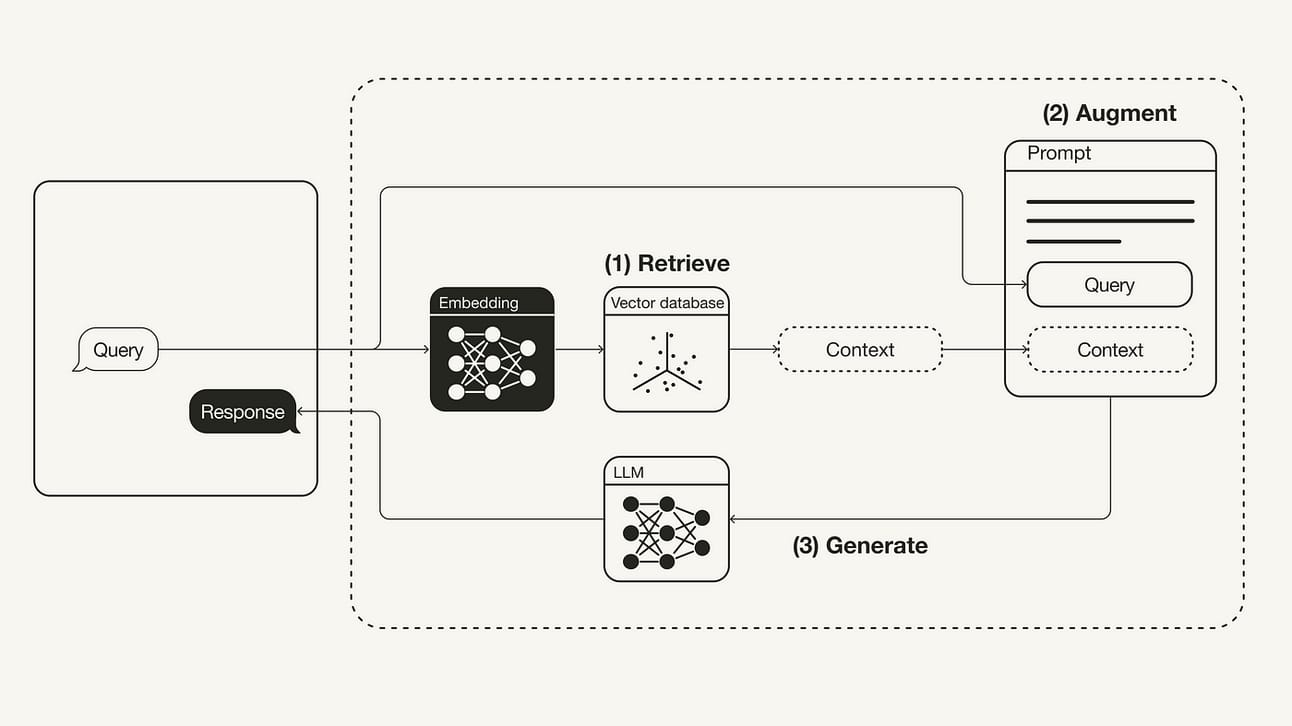
2. Multi-Agent Customer Service & Actions:
-
Often rely on RAG for information retrieval.
-
Can handle complex customer interactions, understand intent, retrieve relevant data, and trigger actions (e.g., issue refunds, send invoices, update CRM).
-
Current Leader:Β n8n (builds upon its RAG capabilities).
Making Your Decision: Which Platform Fits Your Needs?
The choice hinges on your priorities and technical comfort level:
Choose Make.com if you:
-
Prioritize ease of use and a gentle learning curve.
-
Heavily rely on its extensive library of pre-built application integrations.
-
Are building simpler agentic workflows or don’t immediately require RAG/Vector DB capabilities.
-
Value the potential convenience of reusable agent templates.
Choose n8n if you:
-
Require advanced AI agent features like native vector database support for RAG today.
-
Value flexibility, customization, and fine-grained control over agent behavior (memory, tool definition).
-
Need the built-in chat interface for rapid development and testing cycles.
-
Are comfortable working directly with APIs if necessary, but integrations are missing.
-
Benefit from superior subworkflow testing capabilities.
The Future of AI Agents in Automation
Both platforms are on a trajectory of rapid evolution:
-
Feature Convergence: Expect Make.com to close the gap by implementing features currently unique to n8n (like Vector DB support). The core philosophical differences (simplicity vs. flexibility) will likely remain, but the feature sets will become more comparable.
-
Increased Abstraction: The trend is towards simplifying AI development. We may see higher-level interfaces emerge, potentially allowing users to define automation goals using natural language, with the platform handling more of the underlying complexity.
-
Maturation of Standards: Protocols like MCP, if successful, will likely become standard, enabling more seamless integration and interaction between AI models and platform tools across the board.
Conclusion
As of early 2025, n8n holds a demonstrable lead in specialized AI agent functionalities, particularly those reliant on vector databases and offering integrated testing. However, Make.com is a formidable competitor known for rapid development and user-friendliness, and it’s poised to enhance its AI offerings significantly.
The fundamental choice mirrors the long-standing comparison: Make.com offers unparalleled simplicity and integration breadth, ideal for many users. n8n provides greater depth, flexibility, and currently more advanced AI-specific features, appealing to users with more complex requirements or a need for capabilities like RAG now.
Evaluate your immediate needs, technical resources, and tolerance for complexity against the strengths of each platform. The competition is fierce, promising exciting advancements that will ultimately empower users with more intelligent and capable automation tools.
What are your experiences with Make.com or n8n AI agents? Share your thoughts and any key differences you’ve noticed in the comments below!
If you are interested in other topics and how AI is transforming different aspects of our lives, or even in making money using AI with more detailed, step-by-step guidance, you can find our other articles here:
-
The Power of AI Automation: It Can Find and Create Viral Reels for You in Minutes!
-
Quickly Automate Your AI Blogging System: Easy Setup for Instant Success*
*indicates a premium content, if any
Β
Leave a Reply